Why Interpretability is Critical
Interpretable models have many key advantages that streamline the data science process and make it easier to focus directly on deriving value
Understand the strengths and weaknesses of a model
Since an interpretable model is transparent and can be understood in its entirety by humans, we can easily identify whether the model makes sense or not, as well identify parts of the model where the reasoning might be flawed.
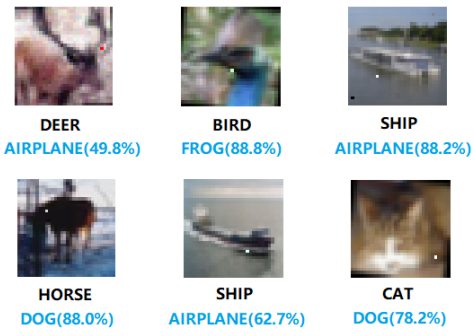
Examples from One Pixel Attack for Fooling Deep Neural Networks. The black text indicates the true label for each image, while the blue text shows the predicted label and confidence in the prediction after altering a single pixel in the image.
Regulatory compliance
Our case study on predicting risk of loan default demonstrates the clarity that interpretability can bring to a complex decision process.
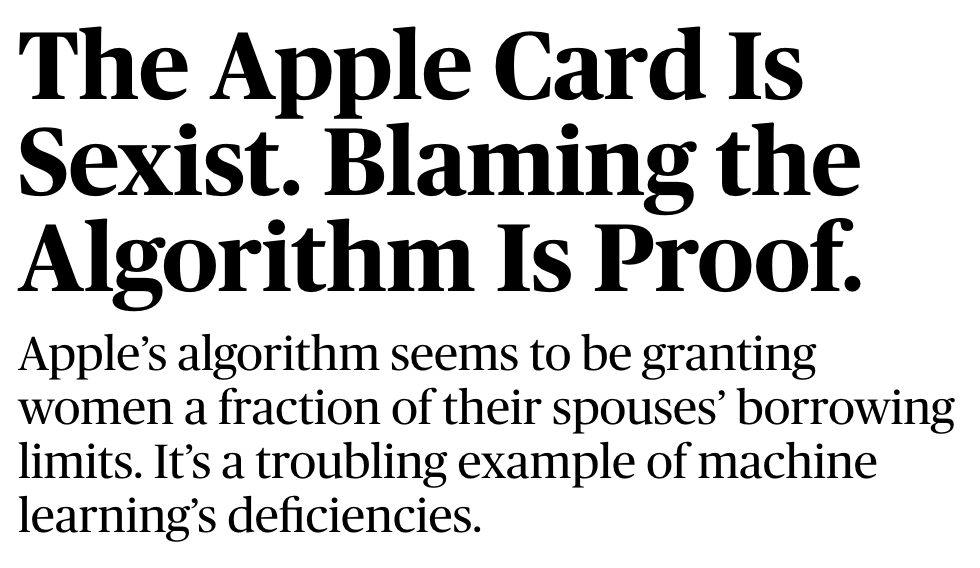
From Bloomberg
Faster iteration and better feedback
In an application to cybersecurity, this rapid iteration enabled our client to capture more relevant data to quickly and directly close holes in the model's reasoning.
The data science process is a continuous iterative loop
Smooth integration of domain expertise
Each of our applications in the medical field rely heavily upon expert doctors providing feedback and ensuring the end result is clinically relevant.
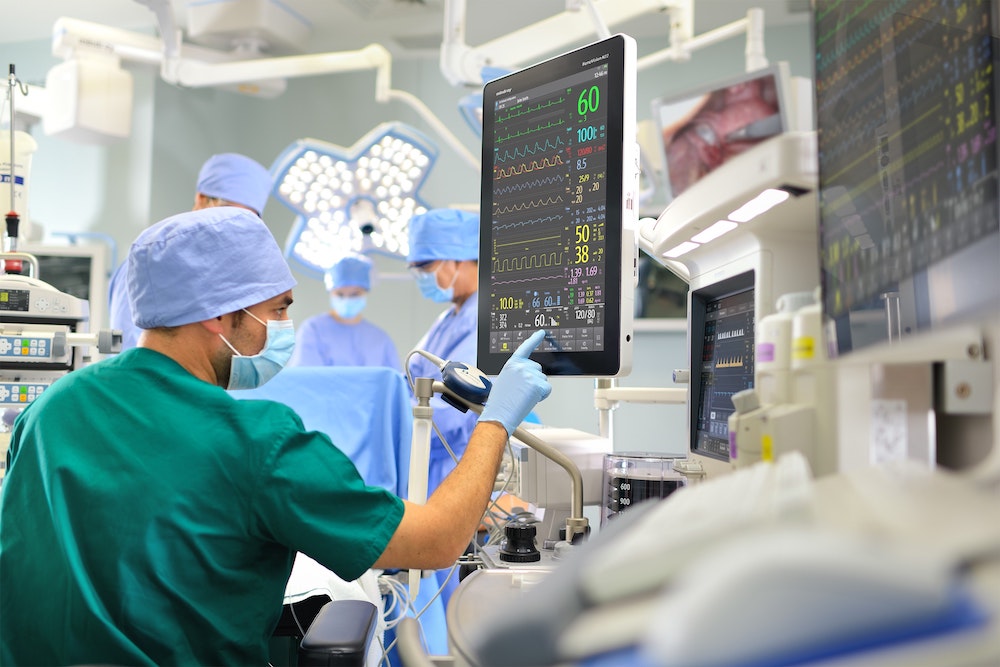
Increased chance of adoption and success
When developing an algorithm for our approach for deciding property prices at auction, we encouraged as many of our clients' portfolio managers as possible to analyze and critique our approach. When it came time to deploy the model the key stakeholders were already on our side and had complete trust in the model due to its interpretability.
If I am going to make an important decision around underwriting risk or food safety, I need much more explainability.
From Artificial intelligence has some explaining to do (Bloomberg)
Learn and discover new insights from data
In a predictive maintenance application, our interpretable models suggested new ideas to the engineers, augmenting their understanding and helping them to prioritize design improvements in order to decrease the machine failure rate.
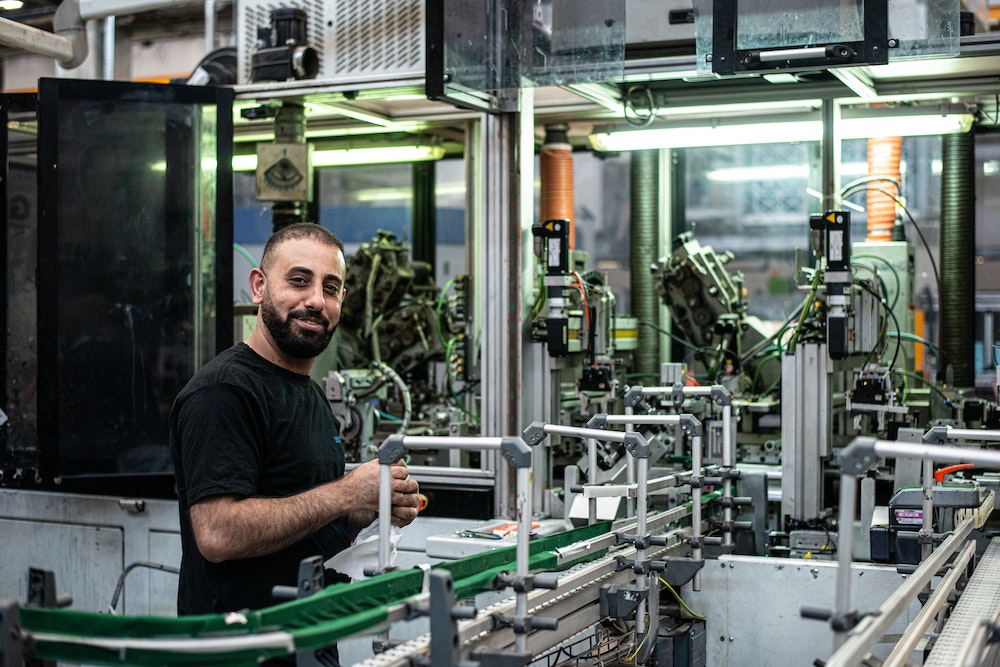
Case studies with interpretable models
Our proprietary interpretable algorithms have been applied successfully in many real-world cases